NLP primers
Confused about NLP? You’re not alone, and it isn’t your fault. Do a Google search on the topic and you will become even more confused.
Wikipedia defines NLP as “a subfield of linguistics, computer science, and artificial intelligence concerned with the interactions between computers and human language, in particular how to program computers to process and analyze large amounts of natural language data.” The article then describes a bewildering array of common NLP tasks that have little relevance to how your company actually works.
Skip the Googling and dig into a few resources we’ve created for business leaders like you:
- Become an AI Company in 90 Days - Our book introduces you to topics like machine learning, training data, and NLP. Take 30 minutes to skim through the relevant sections and you’ll save yourself weeks of research.
- Adventures in AI - The world’s first AI comic book tells the story of how a data scientist uses NLP to save the day at a life insurance company. The story reinforces the value proposition of NLP and the steps to get organizational momentum behind a proof of concept.
- Blog post: The coming NLP Revolution in Insurance - The insurance industry—and life insurance in particular—currently lags behind the rest of financial services in its adoption of information technology. This blog post explains why NLP breakthroughs will fundamentally change the industry in the next few years.
What can NLP do for financial services today?
NLP research is moving at a dizzying pace, but it still has only a handful of capabilities that are mature enough to provide near-term business impact.
Common applications of NLP in financial services
A few NLP technologies have been broadly deployed across many financial services companies. These technologies include tasks like document classification and entity extraction. Machine translation isn’t in this category because most financial services don’t need custom machine learning solutions.
Document classification
NLP is often used to automatically assign a label to sections of unstructured text data. This task is commonly called document classification. Examples:
- Categorizing an entire document based on its contents
- Labeling sections of documents
Entity extraction
Many business processes are based on the nouns referenced within documents. We broadly refer to these nouns as entities: people, places, organizations, products, dates, and so on. Examples:
- Categorizing documents based on specific customers or organizations
- Processing documents to populate graph databases
Promising NLP applications that haven’t delivered
Many NLP technologies get a lot of hype (and sometimes funding), but the business impacts have thus far been elusive. These include natural-language generation (NLG), chat bots, and document summarization.
NLG
The business press has paid a lot of attention to NLG, particularly because of high-profile breakthroughs like GPT-3. Unfortunately the technology isn’t accurate enough to do more than generate simple articles about sports scores or daily stock price movements. For an entertaining example, watch how Russ attempted to use NLG to write the next Game of Thrones book.
Chat bots
Massive investment went into “conversational commerce” a few years ago. But most projects never lived up to expectations. Current chat projects primarily rely on prewritten, canned answers based on rules systems. They don’t use modern NLP techniques.
Document summarization
Wouldn’t it be wonderful if computers could listen to meetings and automatically summarize the conversation for you? That dream is still waiting on the horizon. The technology is still immature, and available solutions only select the most important parts of a document, and the result fall far short of expectations.
How to get a quick win with NLP
Every AI leader is looking for a “quick win,” a way to demonstrate the feasibility of the technology and get further investments. Take a few tips from Prolego–we’ve already worked on dozens of projects in financial services.
Automate tedious reading tasks
Do you devote a lot of personnel to the tasks of reading and sorting documents such as resumes, applications, or call center records? These scenarios are usually opportunities for document classification solutions.
For example, some companies attempt to get support or product insights from reading and processing call center conversations. NLP models might be able to automatically sort and label these conversations according to the business problem.
Stop searching PDFs
A surprising number of lawyers and other highly skilled professionals spend a lot of time searching through PDF documents. After reducing this time sink for several clients, we’ve started referring to it as the “Control-F” problem. Even with the benefit of keyboard shortcuts, PDF searches usually return too many results or too few results. The user is forced to read through the PDF to find relevant information.
This problem is the focus of our comic book, Adventures in AI, volume 1. Lawyers, analysts, and other experts are expensive resources. Any solution that makes their job easier will be a high-visibility success.
Imagine an army of interns
This hack is my secret for finding AI opportunities for any client. It’s especially good at surfacing NLP opportunities.
Not many of your business customers will understand what AI or NLP can do for them. You can help them identify opportunities by using a thought experiment. Just ask them the following question:
Suppose I gave you an army of interns. What would you ask them to do?
An intern is a good proxy for what an NLP model can do. Interns are smart people who can complete some tasks with a modicum of training, even if they have no business context or understanding. You can read more about this hack here.
Improve your graph database
Most financial services companies are beginning to leverage graph technologies to explore complex relationships. A common use case is providing sales, marketing, or support teams a 360-degree view of the customer across all products.
After the basic graph technology is developed, a common constraint is getting accurate data inputs. If your data about customers, products, or needs is contained in unstructured text documents, NLP might be able to extract that information and populate the graph with better data.
Will you survive the NLP revolution?
One of my favorite scenes in Adventures in AI, volume 1, is a discussion about the competitive threat that NLP creates for financial services companies. Lori Santos, the data scientist and hero of the story, is trying to get the VP of Innovation to imagine an NLP-driven future.
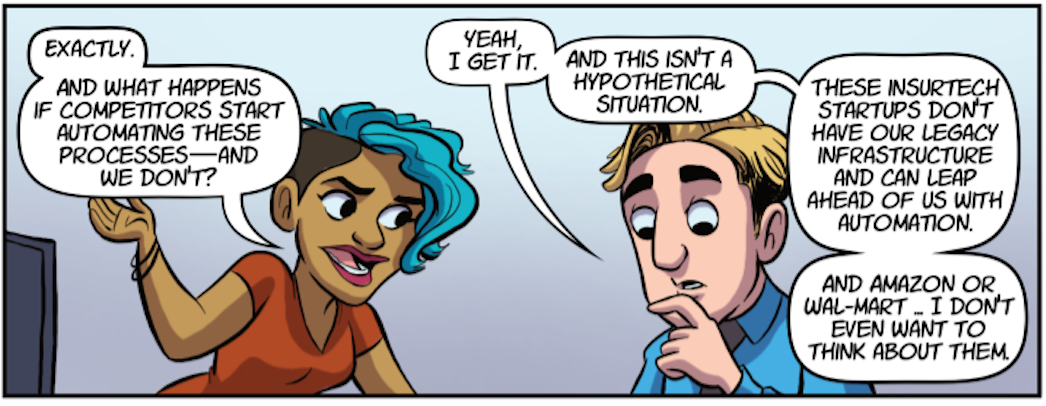
Lori describes what Prolego calls AI Abundance. In this coming reality, AI will be the default operating system that moves the company forward. As part of that transition, NLP will fundamentally change every business process in financial services.
The companies that successfully leverage NLP within the next five years will have a permanent and unassailable advantage in cost and speed. Will you be one of them?